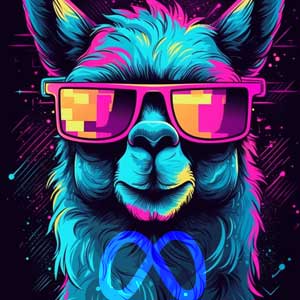
Llama-2 LLM: Versions, Prompt Templates & Hardware Requirements
Explore all versions of the model, their file formats like GGML, GPTQ, and HF, and understand the hardware requirements for local inference.
Meta has rolled out its Llama-2 family of language models, featuring versions with a range of sizes from 7 to 70 billion parameters.. These models, especially the chat-focused ones, perform impressively well against other open-source options and even match some closed-source models like ChatGPT in terms of helpfulness.
The architecture is based on an optimized transformer setup, and models are fine-tuned using supervised techniques and human feedback. They're trained on a vast dataset that doesn't include any user-specific data from Meta.
More about Llama-2
Llama-2 refers to a family of pre-trained and fine-tuned Large Language Models (LLMs) with a scale of up to 70 billion parameters.
Llama 2 underwent its initial training phase using a substantially larger dataset sourced from publicly available online materials, surpassing the dataset size used for its predecessor, LLaMA(1). Following this pretraining stage, Llama-2 Chat was developed through a process of supervised fine-tuning, during which human experts contributed to the training process.
To enhance the model's performance and yield more natural responses, the next stage involved Reinforcement Learning from Human Feedback (RLHF). This method involves an iterative process of refinement, whereby the model is continuously improved through reinforcement learning algorithms and the integration of human feedback.
The Llama 2 family includes the following model sizes:
- 7B
- 13B
- 70B
The Llama 2 LLMs are also based on Google's Transformer architecture, but have some optimizations compared to the original Llama model. These include, for example:
- GPT-3 inspired pre-normalization with RMSNorm,
- SwiGLU activation feature inspired by Google PaLM,
- Multi-Query Attention instead of Multi-Head Attention as well
- Rotary Positional Embeddings (RoPE) inspired by GPT Neo.
The main differences between Llama 2 and Llama are:
- larger context length (4,096 instead of 2,048 tokens)
- trained on larger dataset
- Grouped Query Attention (GQA) instead of Multi Query Attention (MQA) in the two larger Llama-2 models.
Is Llama-2 open source?
Llama 2 is not fully open-source according to the Open Source Initiative's definition because its license imposes restrictions that do not align with the open source criteria. The license restricts commercial use for certain users and purposes, specifically mentioning that services with more than 700 million active monthly users must seek a separate license, potentially excluding major cloud providers. Additionally, the Llama 2 Acceptable Use Policy prohibits using the models for illegal or malicious purposes, which, while understandable, diverges from the open-source principle of unrestricted use.
What is Code Llama?
Code Llama is a variant of the Llama-2 language model, tailored for coding-related tasks. It is capable of generating and completing code, as well as detecting errors in a variety of popular programming languages such as Python, C++, Java, PHP, JavaScript/TypeScript, C#, and Bash. Meta offers Code Llama in three different model sizes: 7B, 13B, and 34B, to cater to different levels of complexity and performance requirements.
Hardware requirements
The performance of an Llama-2 model depends heavily on the hardware it's running on. For recommendations on the best computer hardware configurations to handle Llama-2 models smoothly, check out this guide: Best Computer for Running LLaMA and LLama-2 Models.
Below are the Llama-2 hardware requirements for 4-bit quantization:
For 7B Parameter Models
If the 7B Llama-2-13B-German-Assistant-v4-GPTQ model is what you're after, you gotta think about hardware in two ways. First, for the GPTQ version, you'll want a decent GPU with at least 6GB VRAM. The GTX 1660 or 2060, AMD 5700 XT, or RTX 3050 or 3060 would all work nicely. But for the GGML / GGUF format, it's more about having enough RAM. You'll need around 4 gigs free to run that one smoothly.
Format | RAM Requirements | VRAM Requirements |
---|---|---|
GPTQ (GPU inference) | 6GB (Swap to Load*) | 6GB |
GGML / GGUF (CPU inference) | 4GB | 300MB |
Combination of GPTQ and GGML / GGUF (offloading) | 2GB | 2GB |
*RAM needed to load the model initially. Not required for inference. If your system doesn't have quite enough RAM to fully load the model at startup, you can create a swap file to help with the loading.
For 13B Parameter Models
For beefier models like the Llama-2-13B-German-Assistant-v4-GPTQ, you'll need more powerful hardware. If you're using the GPTQ version, you'll want a strong GPU with at least 10 gigs of VRAM. AMD 6900 XT, RTX 2060 12GB, RTX 3060 12GB, or RTX 3080 would do the trick. For the CPU infgerence (GGML / GGUF) format, having enough RAM is key. You'll want your system to have around 8 gigs available to run it smoothly.
Format | RAM Requirements | VRAM Requirements |
---|---|---|
GPTQ (GPU inference) | 12GB (Swap to Load*) | 10GB |
GGML / GGUF (CPU inference) | 8GB | 500MB |
Combination of GPTQ and GGML / GGUF (offloading) | 10GB | 10GB |
*RAM needed to load the model initially. Not required for inference. If your system doesn't have quite enough RAM to fully load the model at startup, you can create a swap file to help with the loading.
For 65B and 70B Parameter Models
When you step up to the big models like 65B and 70B models (), you need some serious hardware. For GPU inference and GPTQ formats, you'll want a top-shelf GPU with at least 40GB of VRAM. We're talking an A100 40GB, dual RTX 3090s or 4090s, A40, RTX A6000, or 8000. You'll also need 64GB of system RAM. For GGML / GGUF CPU inference, have around 40GB of RAM available for both the 65B and 70B models.
Format | RAM Requirements | VRAM Requirements |
---|---|---|
GPTQ (GPU inference) | 64GB (Swap to Load*) | 40GB |
GGML / GGUF (CPU inference) | 40GB | 600MB |
Combination of GPTQ and GGML / GGUF (offloading) | 20GB | 20GB |
*RAM needed to load the model initially. Not required for inference. If your system doesn't have quite enough RAM to fully load the model at startup, you can create a swap file to help with the loading.
Memory speed
When running Llama-2 AI models, you gotta pay attention to how RAM bandwidth and mdodel size impact inference speed. These large language models need to load completely into RAM or VRAM each time they generate a new token (piece of text). For example, a 4-bit 7B billion parameter Llama-2 model takes up around 4.0GB of RAM.
Suppose your have Ryzen 5 5600X processor and DDR4-3200 RAM with theoretical max bandwidth of 50 GBps. In this scenario, you can expect to generate approximately 9 tokens per second. Typically, this performance is about 70% of your theoretical maximum speed due to several limiting factors such as inference sofware, latency, system overhead, and workload characteristics, which prevent reaching the peak speed. To achieve a higher inference speed, say 16 tokens per second, you would need more bandwidth. For example, a system with DDR5-5600 offering around 90 GBps could be enough.
For comparison, high-end GPUs like the Nvidia RTX 3090 boast nearly 930 GBps of bandwidth for their VRAM. The DDR5-6400 RAM can provide up to 100 GB/s. Therefore, understanding and optimizing bandwidth is crucial for running models like Llama-2 efficiently
Recommendations:
- For Best Performance: Opt for a machine with a high-end GPU (like NVIDIA's latest RTX 3090 or RTX 4090) or dual GPU setup to accommodate the largest models (65B and 70B). A system with adequate RAM (minimum 16 GB, but 64 GB best) would be optimal.
- For Budget Constraints: If you're limited by budget, focus on Llama-2 GGML/GGUF models that fit within the sytem RAM. Remember, while you can offload some weights to the system RAM, it will come at a performance cost.
Remember, these are recommendations, and the actual performance will depend on several factors, including the specific task, model implementation, and other system processes.
CPU requirements
For best performance, a modern multi-core CPU is recommended. An Intel Core i7 from 8th gen onward or AMD Ryzen 5 from 3rd gen onward will work well. CPU with 6-core or 8-core is ideal. Higher clock speeds also improve prompt processing, so aim for 3.6GHz or more.
Having CPU instruction sets like AVX, AVX2, AVX-512 can further improve performance if available. The key is to have a reasonably modern consumer-level CPU with decent core count and clocks, along with baseline vector processing (required for CPU inference with llama.cpp) through AVX2. With those specs, the CPU should handle Llama-2 model size.